Overarching Goal
The overarching goal of the project was threefold. First, we integrated digital imaging into Temple University Hospital’s clinical operations. Then, we established a public database of pathology slides. Lastly, we developed an image classification system to automatically annotate each pathology slide. The result was a fully functional digital pathology imaging system. After the database of pathology slides were annotated using this system, they were archived and used for support, education, and/or research purposes.
Past Goals
- Integrated digital imaging into Temple University Hospital's clinical operations.
- Created a database of pathology slides.
- Developed an image classification system that automatically annotates each slide.
- As a result, a fully functional digital pathology imaging system was developed.
Current Goals
- Combine image data extracted from a digital slide scanner with text data extracted from medical reports.
- Create and maintain a pathology knowledge graph.
- Linking images to text in reports.
- Create a Visio-and-Language Retrieval System.
Future Goals
- Create a retrieval system that works in a traditional fashion where a user supplies her query via mouse or keyboard. Eventually, we hope to create a conversational-based retrieval system.
- We plan to release more than one million images.
Anticipated Outcomes
First, the open-source unencumbered corpus that we will
develop will be released to the community to support machine
learning research. The Neural Engineering Data Consortium
(NEDC)
has been in existence since 2012 and is known internationally for
distributing the world’s largest open-source corpus, the TUH EEG
Corpus (TUEG), to support machine learning research in
electroencephalography (EEG).This corpus currently has over 4,000
subscribers.
Second, we will develop two robust systems: (1) an image processing
system that rapidly flags tissue samples that are cancerous or
abnormal, and (2) a text processing system that identifies key
medical concepts and relationships in reports and integrates this
information along with events identified in the images into a
searchable archive.
Third, we will develop an interactive tool that pathologists can use
to query the database using natural language queries and form-filling.
The ability to retrieve slides from a flexible query interface is
very important to clinicopathologic correlative studies, which are
critical for the study of the clinical pathologic features, treatment
response and prognosis of uncommon diseases. The existing query
methods in most pathology software are inefficient.
Timeline
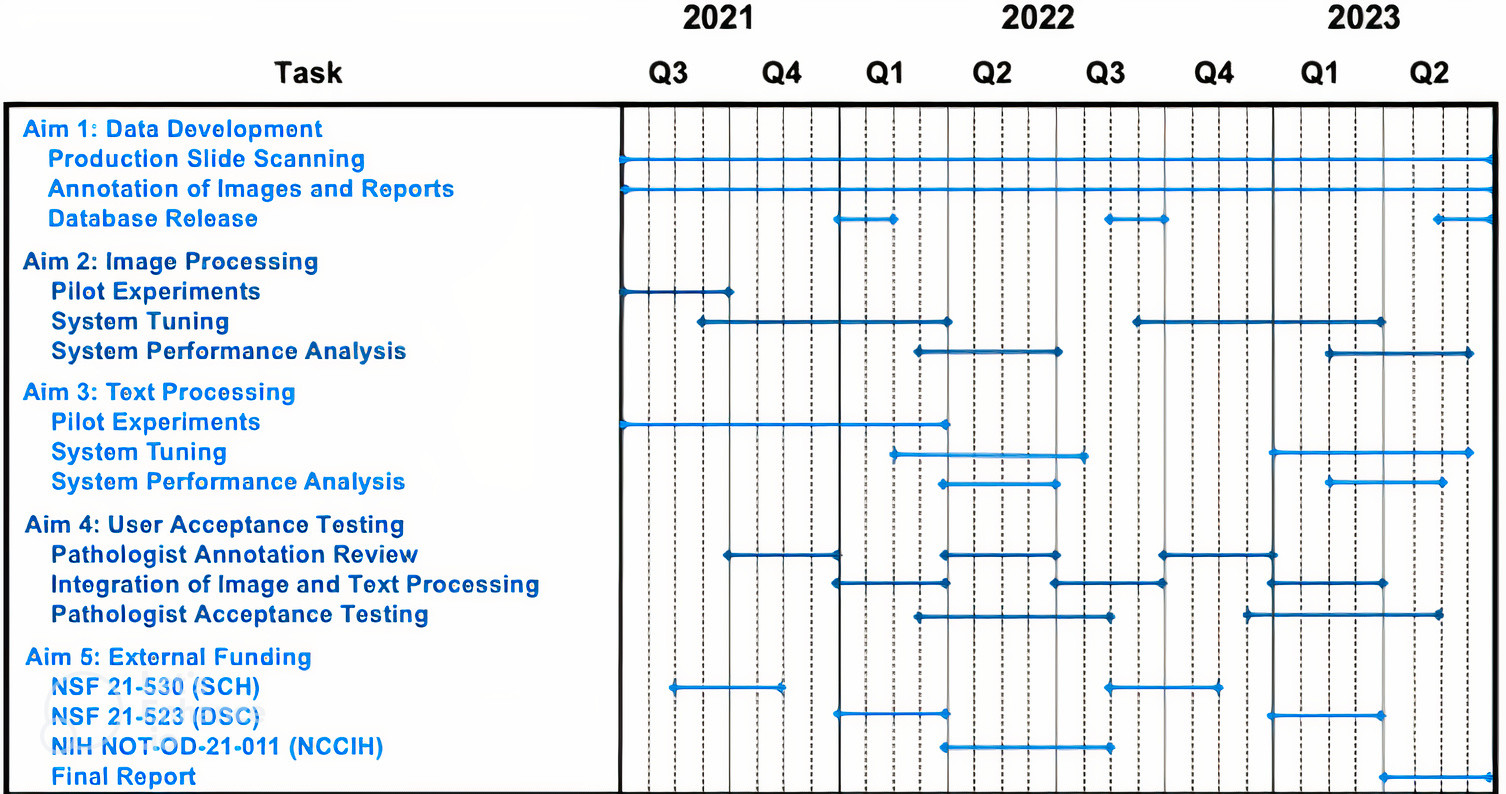