Automatic Interpretation of Digital Pathology Images Using Deep Learning
In this NSF-funded project, we are developing a digital imaging system using big data and machine learning algorithms to automatically characterize pathology slides. We have developed a sustainable facility to rapidly collect automatically annotated slide images. This project has produced the necessary data resources to support the development of high performance deep learning models.
Overview
We had proposed a collaborative and interdisciplinary project to
detect and characterize cancerous cells in digitized images of
pathology slides, while also producing the world's largest catalog
of research-grade digital pathology slides.
The research allowed our uniquely qualified team, which spans the
disciplines of pathology, engineering and computer science, to pursue
center-level investments from NSF and NIH.
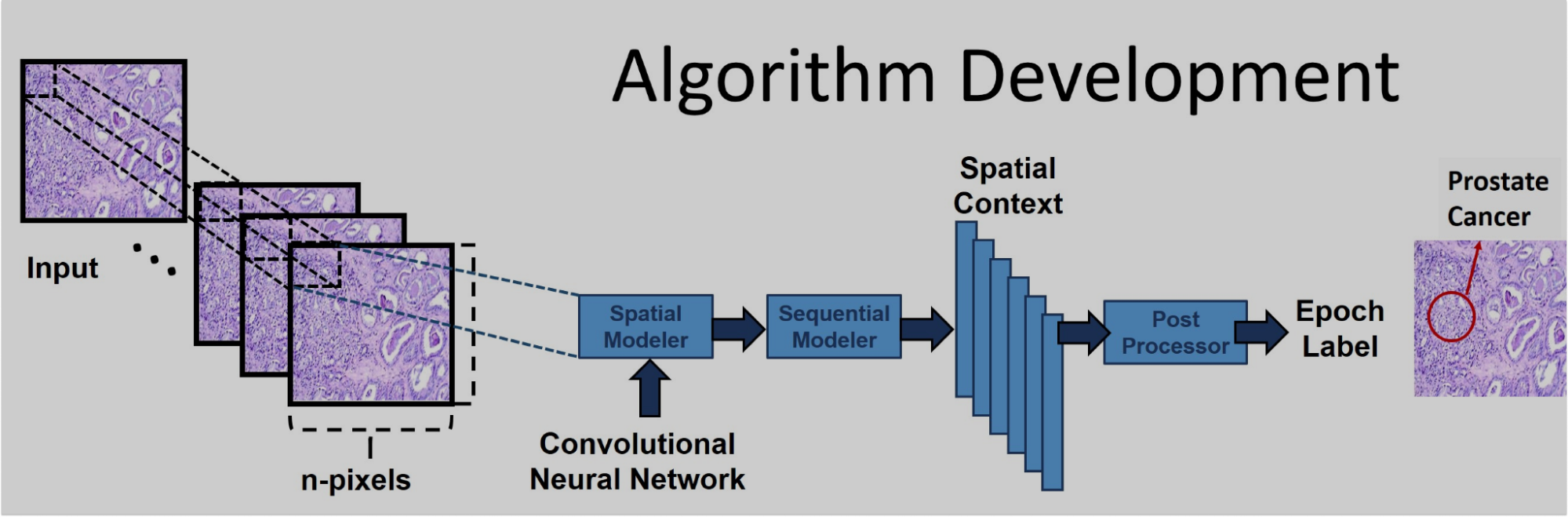
Over 10 million slides are read each year in the U.S. alone. Tapping into a fraction of this data allows significant advancement of the science. Healthcare providers and machine learning researchers will be able to access an open source high-quality searchable archive of clinical data. More information on this project can be found here.
Goals
Our initial goal will be to combine image data extracted from a digital slide scanner with text data extracted from medical reports. Pathology reports contain unstructured text data that describe patient histories, medications and diagnoses.
We are developing our database, processing high-resolution digital pathology images, and performing integrated text processing using Visio-and-Language queries.