What's New
(20240921) We have released v1.0.0 of the FCCC Digital Pathology Corpus (FCDP). This release contains over 14,000 images, including 1,400+ annotated images of breast tissue. Go here to learn more.
(20240520) We have migrated our DPATH resources to a central location here where you will find all our data releases. Over the next few weeks we will be releasing several very large DPATH datasets.
(20211215) We have completed scanning the Fox Chase Cancer Center subset and now have scanned over 120,000 slides.
Project Summary
In this NSF-funded project, we are developing a digital
imaging system using big data and machine learning algorithms
to automatically characterize pathology slides. We have
developed a sustainable facility to rapidly collect
automatically annotated whole slide images. This project is
producing the necessary data resources to support the
development of high performance deep learning models.
Over 10M slides are read each year in the U.S. alone. Tapping
into a fraction of this data will allow significant
advancement of the science. Healthcare providers and machine
learning researchers will be able to access an open source
high-quality searchable archive of clincial data. More
information on this project can be found
here.
A Cost-Effective Image Management Platform
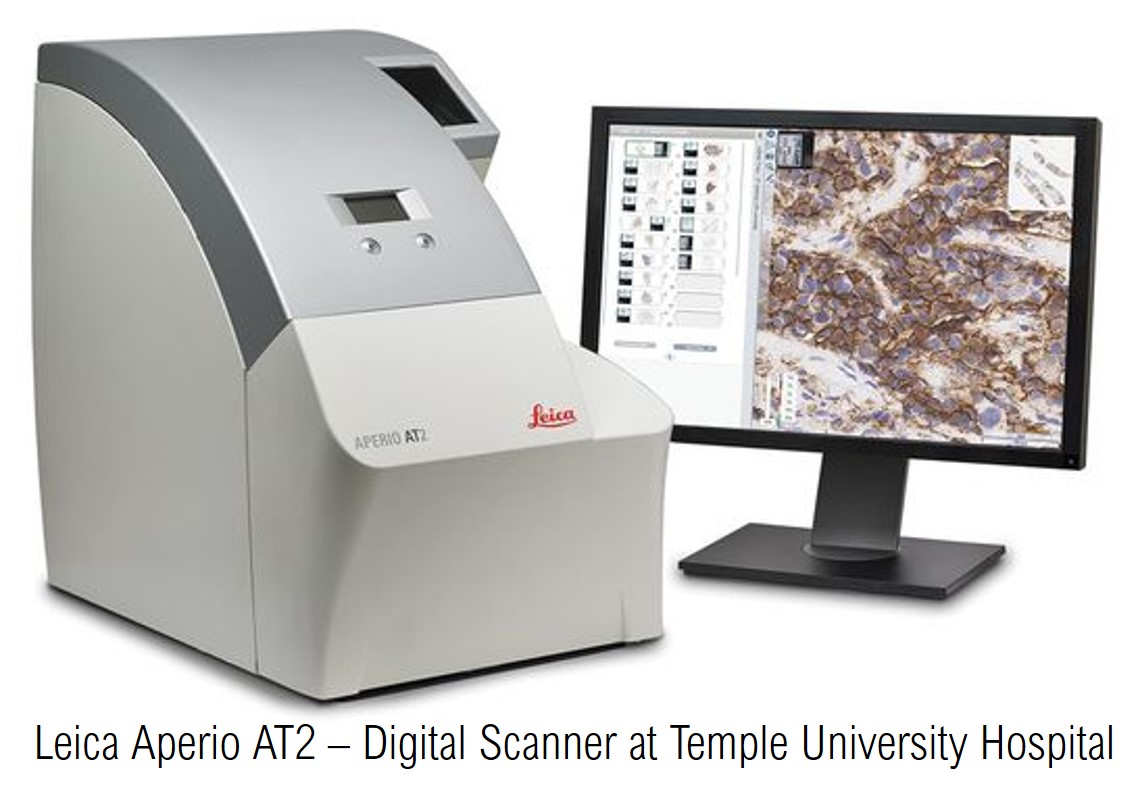
This NSF Major Research Instrumentation (MRI) grant supported
the purchase of a
Leica Aperio AT2
scanner as the platform used to convert pathology slides to
digital images. This scanner can scan 50 high quality TIFF images
with lossless compression per hour.
We have also developed a very cost-effective Petabyte file store
based on off-the-shelf components to store our databases.
To learn more about our clustered computing
environment being developed to support this research program,
read this
overview.