Significance
Data-driven approaches have made enormous advances in recent years in terms of their ability to predict events through supervised training on big data resources. Equally important, however, is the fact that many of these techniques have the ability to discover underlying structure of the data using latent variables and unsupervised training techniques. These types of algorithms can provide enormous insight into the data. The only impediment to applying these techniques has been the lack of a suitable amount of data to support comprehensive experimentation. The Neural Engineering Data Consortium (NEDC) has been established at Temple University to address this issue.
Goal
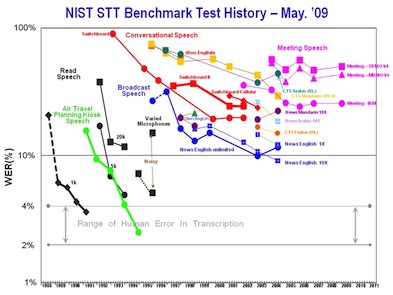
The existence of massive corpora has proven to substantially accelerate research progress, as shown to the right. NEDC will broaden participation by making data available to research groups who have significant signal processing expertise but who lack capacity for data generation. This effort is modeled in part after similar successful endeavors, particularly in the human language technology field where a data consortium has led to systematic research and technology advances over a 20 year span.
The TUH EEG Corpus represents NEDC's first effort to provide a massive corpus to the community. In this project, we are focusing on clinical electroencephalogram (EEG) recordings, as shown to the left. These recordings were conducted at Temple University Hospital (TUH) from 2002 to 2013 (and beyond). The first release of the corpus is expected to contain over 20,000 EEGs. We expect to continue the development of the corpus at a rate of about 1,000 EEGs per year. We also hope to convince other hospitals to provide similar data. The end goal for the corpus is over 100,000 EEGs.
Project Distinction
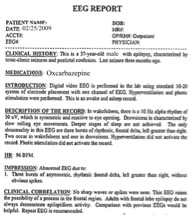
What makes this corpus unique is that in addition to the signal data, for each EEG, a physician's EEG Report is available. An example of such a report is shown to the left. This report contains a summary of the patient’s clinical history and medications. It also includes two fields, Impression and Clinical Correlation, which contain the physician's findings. This report information is available in an Excel spreadsheet in a name/value pair format. The more recently collected EEGs (since 2011) are also coded in International Statistical Classification of Diseases codes (ICD 9). These codes can form the basis for the classification labels used in machine learning experiments
Such a large corpus will support the development of technology to automatically interpret EEGs in addition to advancing the basic science of what aspects of a patient's medical record correlate with various pathologies that can be diagnosed from EEG studies. Automatic interpretation of EEG data using machine learning approaches has evolved in recent years. Several specific applications have been studied extensively, including seizure detection, movement and brain activity. What most of these studies have in common, however, is that the data sets are small, typically involving 100 or less EEG studies. Such small studies simply do not produce statistically significant outcomes, and do not represent enough data to support complex statistical models. Further, when correlates such as drug treatments, patient medical histories, or patient gender or age are factored in, studies consisting of 100 subjects are not sufficient to draw conclusions about best practices. It is the goal of this project to fundamentally change this.
Electroencephalography
Electroencephalography (EEG) is the recording of electrical activity along the scalp using an array of electrodes positioned strategically around a patient's head. For an excellent tutorial on the hardware aspects of this problem, see E. Pavlick's EEG Tutorial. EEGs are used today to diagnose many problems ranging from epilepsy to sleep disorders. The EEGs contained in the TUH EEG Corpus consist of 24 to 36 channels of signal data and an annotation channel containing markers identifying events of interest to the physicians and technicians. Signals are sampled at 250 Hz using 16 bits per sample. A typical EEG file contains about 20 Mbytes of data. These files are stored in an European Data Format (EDF+) file format.
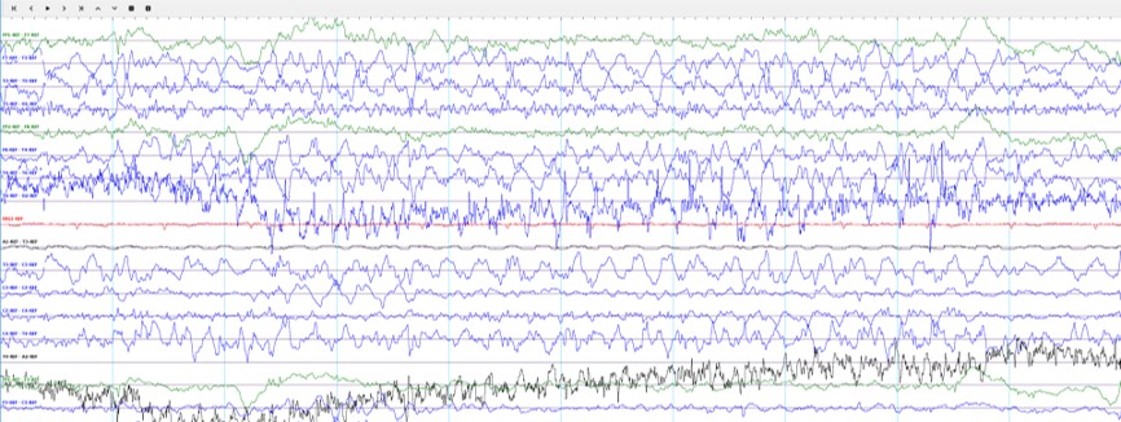