Goal
Our goal is to quadruple the size of the TUH EEG Seizure Detection Corpus by manually annotating three years’ worth of EEG data collected at Temple University's Hospital. This additional data, to be released into the public domain, will allow us to improve our seizure detection technology, enhance a commercialization effort, as well as strengthen a significant research contribution to the field.
Background Information
An EEG (electroencephalogram) records electrical activity along the scalp. The entire session for a routine EEG, including the time required to affix sensors to a patient’s scalp, requires one to two hours. Patients are asked to lie still in a prone position, and are periodically requested to perform limited movements (e.g., breath, blink). Patients who experience medical conditions such as epilepsy or stroke are often subjected to long-term monitoring in a critical care setting such as an Epilepsy Monitoring Unit (EMU). In such cases recordings can last several hours or several days, generating a large amount of data that needs to be reviewed by a clinician. Unfortunately, the data collected under such conditions is often sufficiently noisy and poses serious challenges for automated analysis systems.
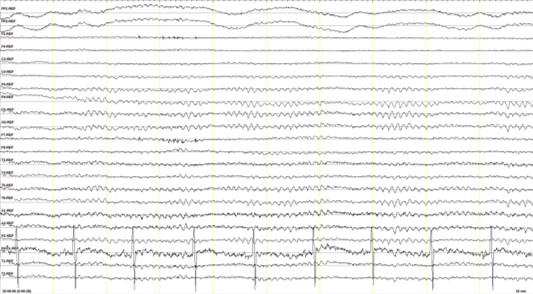
The increasing scope of conditions addressable by EEGs suggest that there is a growing need for expertise to interpret them and, equally importantly, research to understand how various conditions manifest themselves in an EEG signal. Computer-generated EEG interpretation and indentification of critical events can thererefore be expected to significantly increase the quality and efficiency of a neurologist’s diagnostic work. Clinical consequences include regularization of EEG reports, real-time feedback and decision-making support to physicians. Computerized EEG assessment can therefore potentially alleviate the bottlenecking of inadequate resources to monitor and interpret these tests.
Significance
State of the art seizure detection algorithms currently have false alarm rates of 50 per 24 hours with a latency of 10 seconds or more. The data developed through PA CURE Seizure will allow deep learning algorithms to reduce error rates by an order of magnitude or more, making the technology more viable in clinical settings. This will enable improved care in critical care settings as neurologists will be able to monitor patients in real-time rather than treat them retrospectively. Additionally, with access to reliable real-time alerts, healthcare providers will be able to efficiently monitor multiple patients in large critical care settings.
Project Description
The evaluation set for this corpus consists of 50 patients while the
training set consists of 250 patients. Seizure event annotations
include: start and stop times; localization of a seizure (e.g., focal,
generalized) with the appropriate channels marked; type of seizure
(e.g., simple partial, complex partial, tonic-clonic, absence, atonic);
and the nature of the seizure (e.g., convulsive). The non-seizure event
annotations include: artifacts which could be confused with seizure-like
events such as ventilatory artifacts and lead artifacts;
non-epileptiform activity that may resemble epileptiform discharges,
such as psychomotor variant, mu, breach rhythms and positive occipital
sharp transients of sleep (POSTS); abnormal background which could be
confused with seizure-like events (e.g. triphasics); and postictal
stages. The types of features are important when manually interpreting
an EEG and determining how a seizure manifests itself. These finer
categorizations of seizures are used to build models specific to
these events, which reduces the false alarm rate.
Annotations are developed by a team consisting of a graduate student
and trained undergraduates. This team has been in place for almost one
year and has been producing high quality work. Standard
cross-validation approaches are used to ensure that the data produced
by the annotation team is consistent and accurate. Thus far, inter-rater
agreement has been very high (Kappa statistics above 0.8). Inter-rater
agreement amongst neurologists tends to be lower (Kappa statistics on
the order of 0.5).
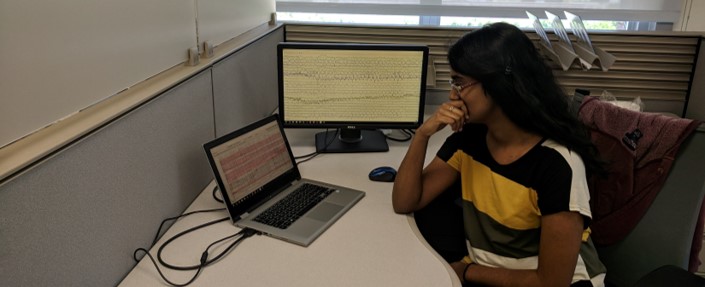
A fairly rigorous process has been used to automatically locate data with a high probability of containing a seizure. This is done using a mixture of Neural Engineering Data Consortium technology, some commercially available technology, and a probabilistic modeling process that looks for the intersection of a number of outputs from these systems. Therefore, data needing manual review is reduced by two orders of magnitude. The resulting files are then manually processed by the annotation team at a rate of approximately 10xRT (10 times real-time). Therefore, every minute of data takes 10 minutes of annotator time to resolve. The annotation team produces data at least 10x faster than expert neurologists at approximately 1/100 the cost; yielding results that are more than acceptable for deep learning systems.