EEG Annotation Workflow
In our project, annotators process approximately 10 files (equivalent to 5 hours of EEG data) per hour. Following initial annotation, a project manager reviews the data, selecting specific files for further scrutiny based on observed patterns and potential inconsistencies. This review process helps ensure the quality and consistency of the annotations across the dataset.
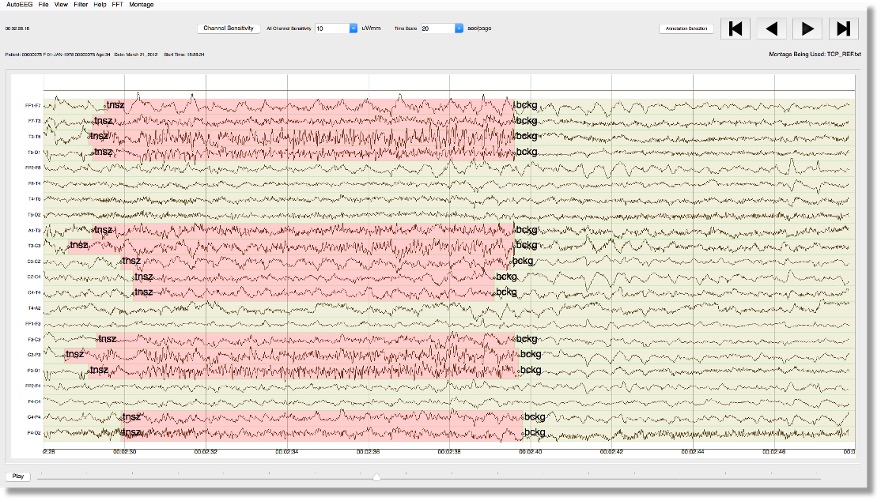
To further enhance the annotation process, we utilize the NEDC EEG Annotation System, an EEG system trained on TUEG data. This system automatically triages data by identifying segments with potential seizure activity, significantly reducing manual review time. The underlying ResNet-based architecture provides precise analysis of EEG signals, allowing us to efficiently label complex data while maintaining accuracy.
In addition to automated support, our team holds weekly meetings to discuss particularly challenging cases. These discussions facilitate consensus on complex annotations and help standardize labeling practices across the team. This collaborative approach, combined with the use of advanced tools, ensures both high productivity and the reliability of our EEG annotations.
Seizure Analysis
Shivering is often associated with underlying neurological activity, sometimes showing ictal patterns in the EEG. However, in this case, the absence of spike-and-wave activity suggests that the observed shivering is not related to seizure events, highlighting the need to differentiate between various neurological responses.
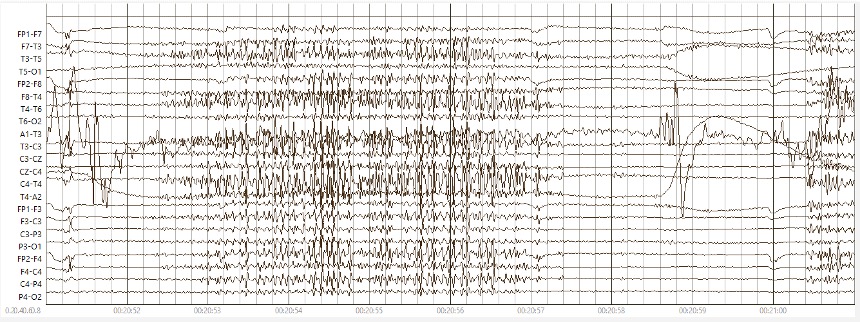
Seizures in patients with Lennox-Gastaut syndrome (LGS) are challenging to identify, as they often occur against a background of slow spike-and-wave activity, interspersed with intermittent bursts of sharp spikes. This complex pattern complicates the detection of seizures, requiring careful analysis to distinguish them from the baseline EEG activity.
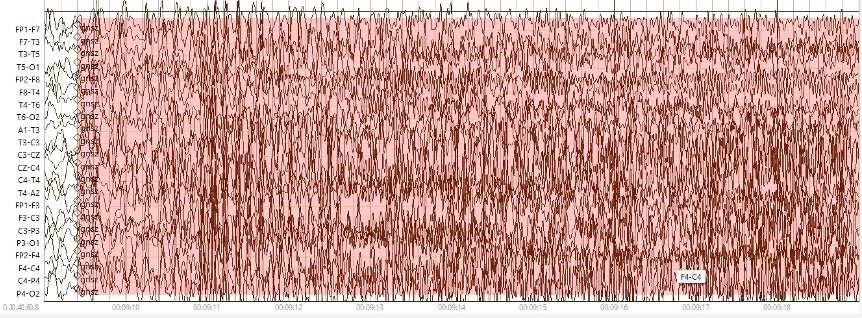
Future Work
As part of our ongoing efforts, future work will focus on annotating Rapid Population Potentials (RPPs) and Burst Intermittent Rhythmic Discharges (BIRDs), which will be pivotal for advancing the next generation of seizure prediction models. These annotations will allow us to capture a broader range of EEG activity and better understand its relationship to seizure onset, providing valuable insights to improve prediction accuracy.
We also plan to train machine learning models on continuous EEG recordings that include both seizure and RPP annotations. This will enable us to build more robust and accurate predictive algorithms by utilizing larger, more diverse datasets. Specifically, we will train our models using the TUEG and NMAE corpora, which will enhance our model's ability to generalize across different datasets.
One of the key challenges we are addressing is understanding how our model makes decisions when annotating seizure events. At present, we have not observed a clear pattern in how the model identifies and classifies seizures. To overcome this, we intend to further investigate the functionality of the decoder, with the goal of improving its interpretability. This will be crucial for enhancing the transparency of the model and ensuring its reliability for clinical use.