Transformers have significantly improved accuracy in image analysis, particularly in digital pathology. This work focuses on two advanced models, including a baseline CNN architecture (ResNet-18). While CNNs have been dominant in visual AI, Vision Transformers (ViT) show promise in capturing long-range dependencies through self-attention mechanisms. For our upcoming software version we are currently exploring numerous advancements including automatic segmentation as well as a transformer-based classifier which are under evaluation for their potential impact.
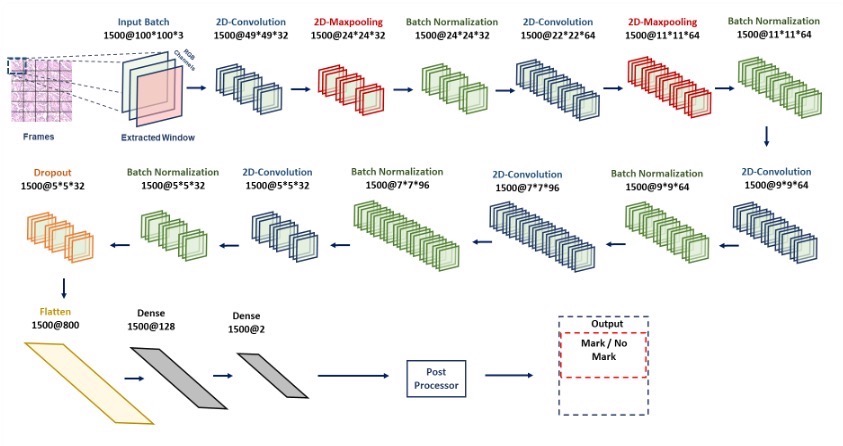
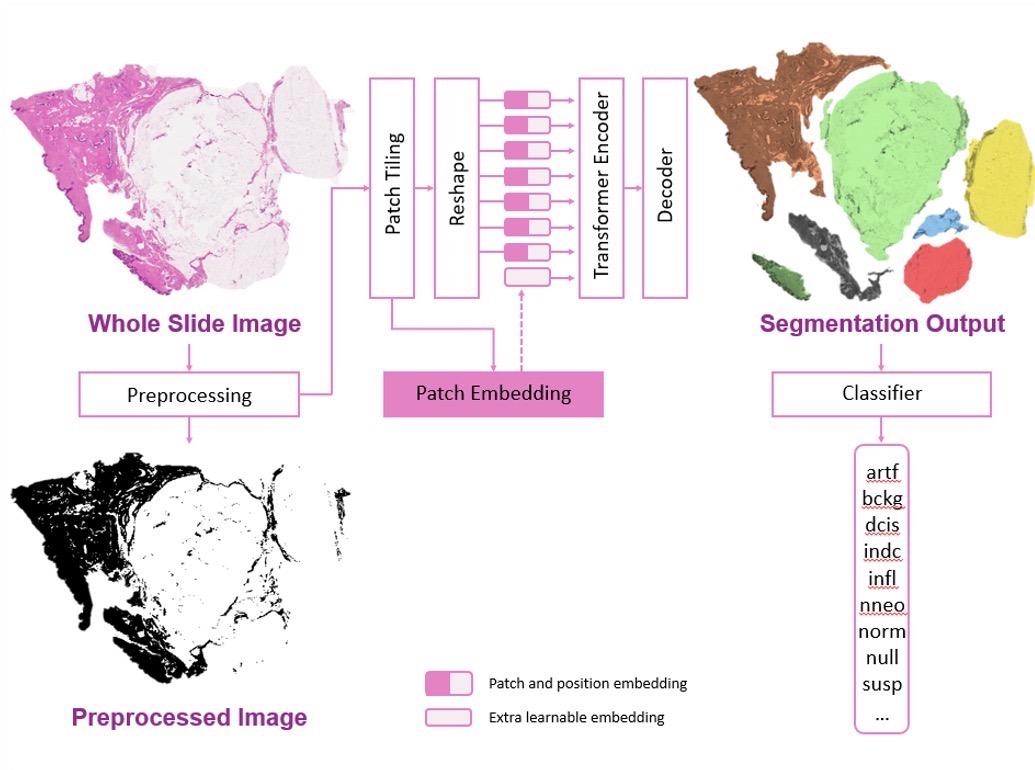
Pathology slides are digitized using a Leica Aperio AT2 scanner located at Temple University Hospital (Room A2-F322). This advanced scanner can hold up to 400 slides, organized into 10 carousels with 40 slides each. While the scanning process is efficient, it does require manual intervention, particularly in adding focus points to ensure optimal image clarity for pathology analysis. On average, the scanner achieves a scanning rate of about 50 slides per hour, allowing for the complete digitization of 400 slides in approximately 8 hours. This combination of high capacity and precision facilitates a streamlined workflow for quality image acquisition in pathology.
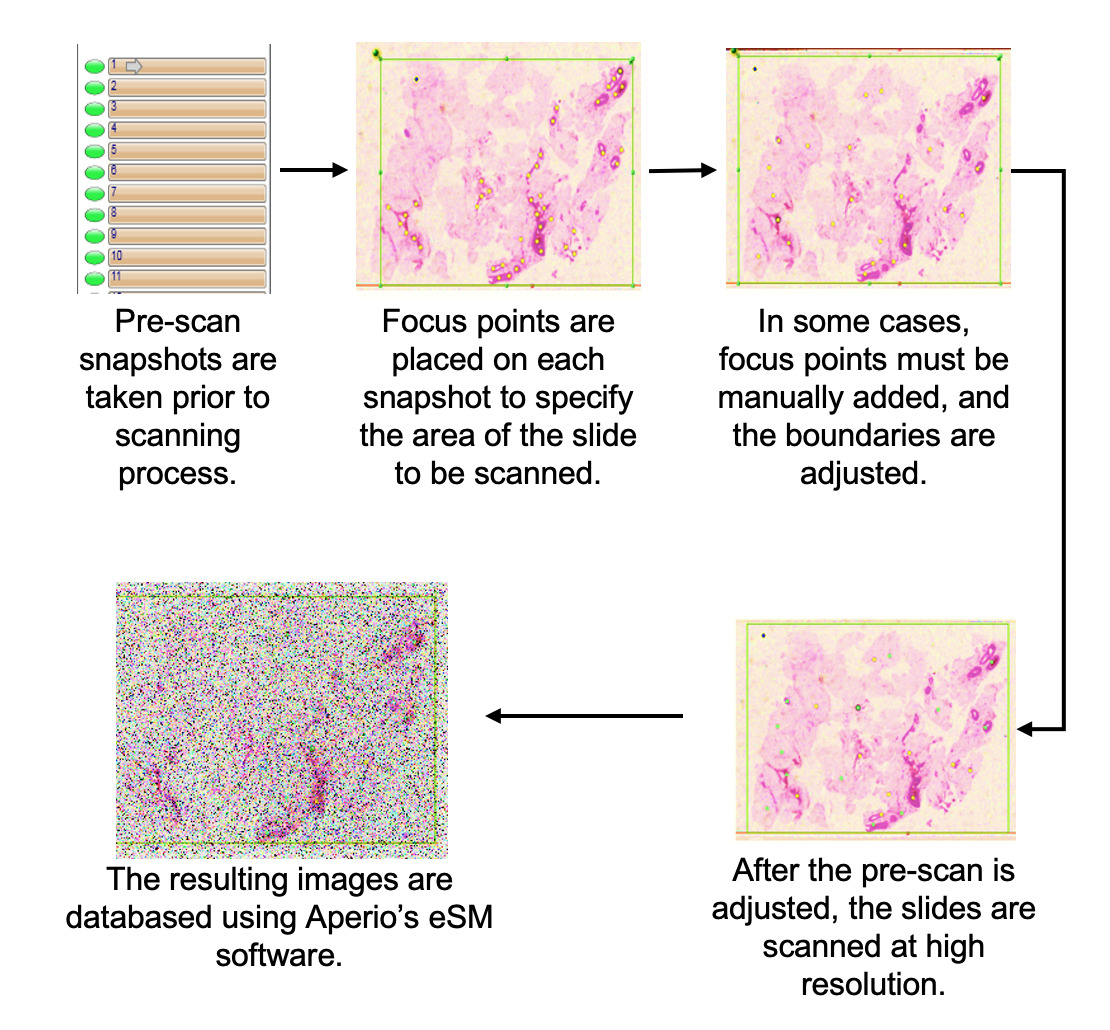
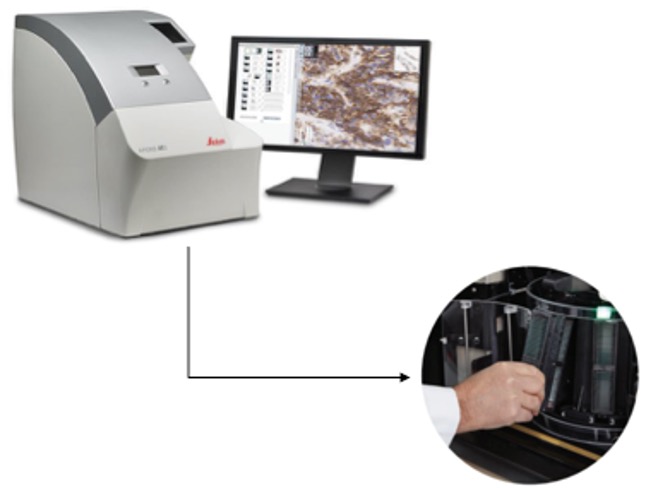
We have digitized over 100,000 slides, including data from various sources such as the Temple archives (TUH), Temple Tumor Board data (TUH-TB), and the Fox Chase Cancer Center Biosample Repository (FCCC). Currently, we are in the process of renaming and de-identifying these slides to align with our database format, which involves a manual review of the slide information. In addition to the slides, we also capture and de-identify pathology reports. Our current focus is on the annotation of the breast tissue subset of the FCCC data, which will complement our previously released TUHS Breast Tissue subset, consisting of 3,505 annotated slides.
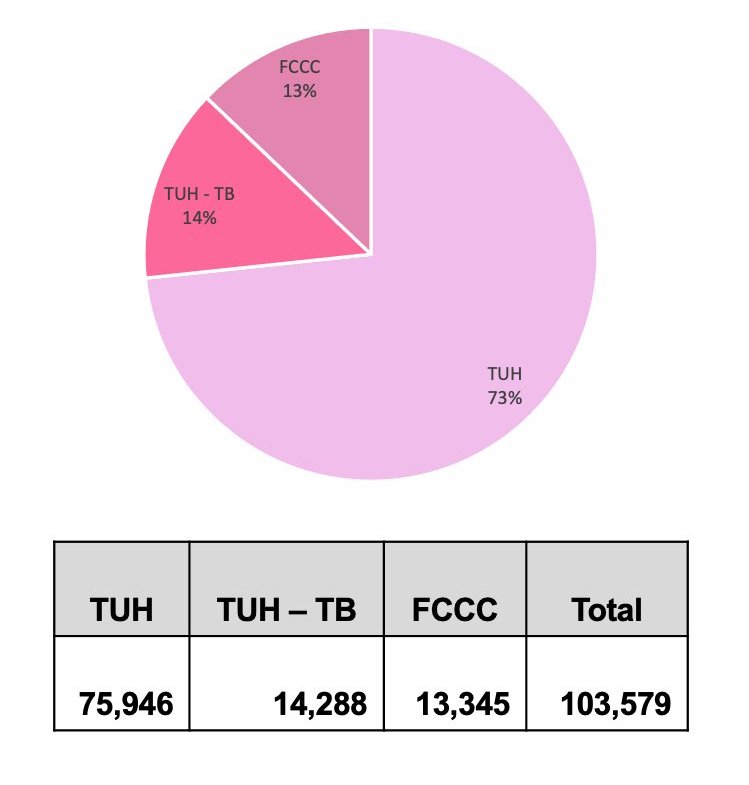
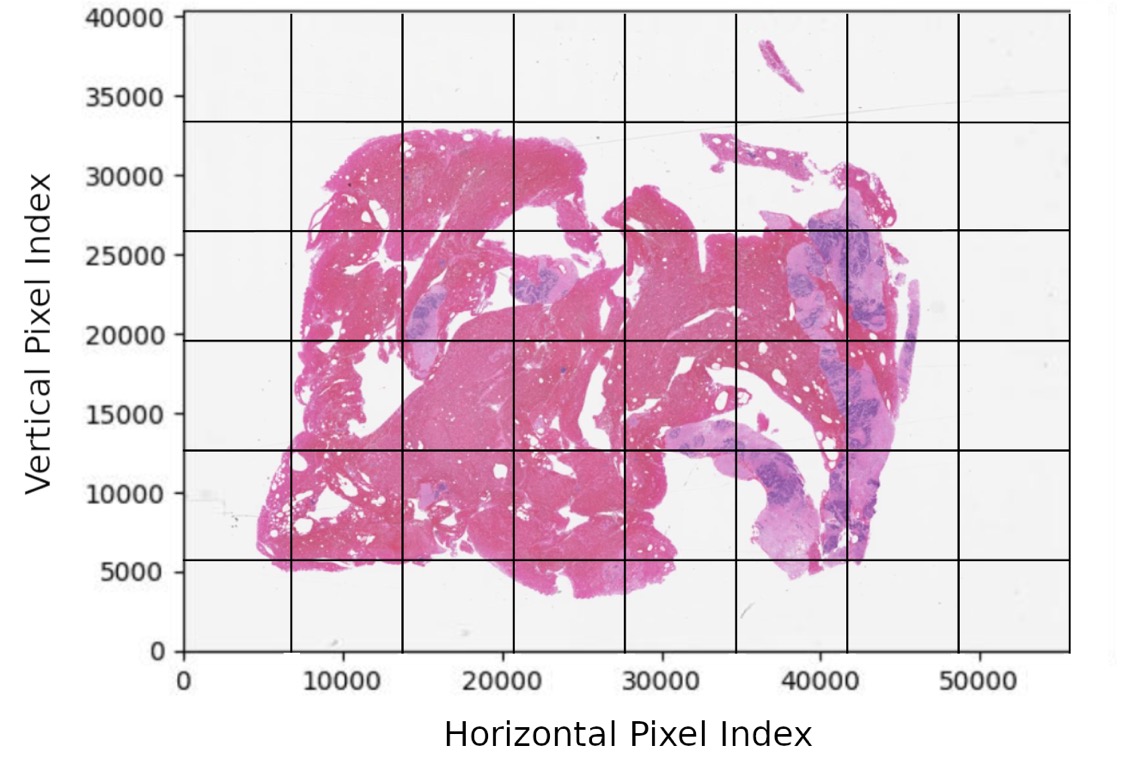